date
type
status
slug
summary
tags
category
icon
password
URL
July 26, 2021 • 2 min read
by Simon's Daydream, mp.weixin.qq.com • See original
In this year’s CDRF 2021 (Tongji Digital Futures 2021 Conference), I presented my paper titled "Architectural Facade Perpetual Motion Machine — Exploring Architectural Design in High-Dimensional Latent Space" (translated title, casually translated to make it sound impressive). This Chinese explanation video explores an AI method capable of generating high-confidence architectural facades and visualizing images in high-dimensional latent space based on their latent vector coordinates, along with performing semantic operations on image content using principal component analysis.
Some advanced mathematics and machine learning concepts will be discussed; feel free to ask me questions if you don’t understand (though I might not be able to explain it any clearer!). I am not from a computer science background, so if there are any mistakes, please feel free to point them out gently!
Demo
Explanation
1 Introduction
With the emerging of Generative Adversarial Network (GAN) based image generation methodin recent years, many attempts havebeen made to apply GAN into architectural images and drawing generation research(Goodfellowet al. 2014). However, for therealistic building façade images generation task, most attempts faced different challenges, such as quality and controllability ofgenerated image, and interpretabilityof model.
These challenges were due to various limitations, such as performance ofthe selected GAN model, the size of training dataset, the understanding oflatent space, etc. In this paper, by training the state-of-the-art GAN basedimage generation model, StyleGAN2 (Karras et al. 2020), with high-resolution building façade image dataset, andexploring its latent space by applying PCA and GANSpace analysis, we couldovercome above challenges in different extend (Härkönen et al. 2020).
In summary,the main functions and contributions of this paper are:
1. A StyleGAN2 model instance which could generate plausible buildingfaçade images without conditional input.
2. Introduce GANSpace and image embedding method to visualize the correlationbetween the generated building façade images and their corresponding latentvectors, which achievedunsupervised classification and high-level propertiescontrol of both generated and novel images.
- 作者:Simon Shengyu Meng
- 链接:https://simonsy.net/article/stylegan-arch-en
- 声明:本文采用 CC BY-NC-SA 4.0 许可协议,转载请注明出处。
相关文章
DreamGaussian: The Stable Diffusion Moment of AIGC 3D Generation
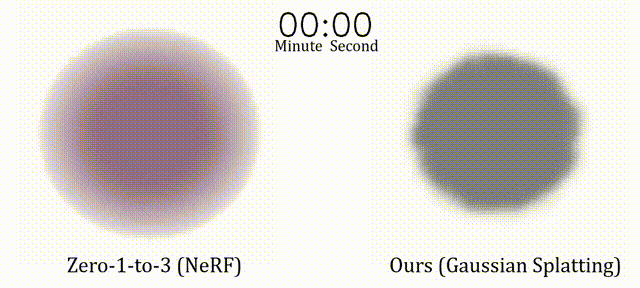
How I Used AI to Create a Promotional Video for Xiaomi's Daniel Arsham Limited Edition Smartphone
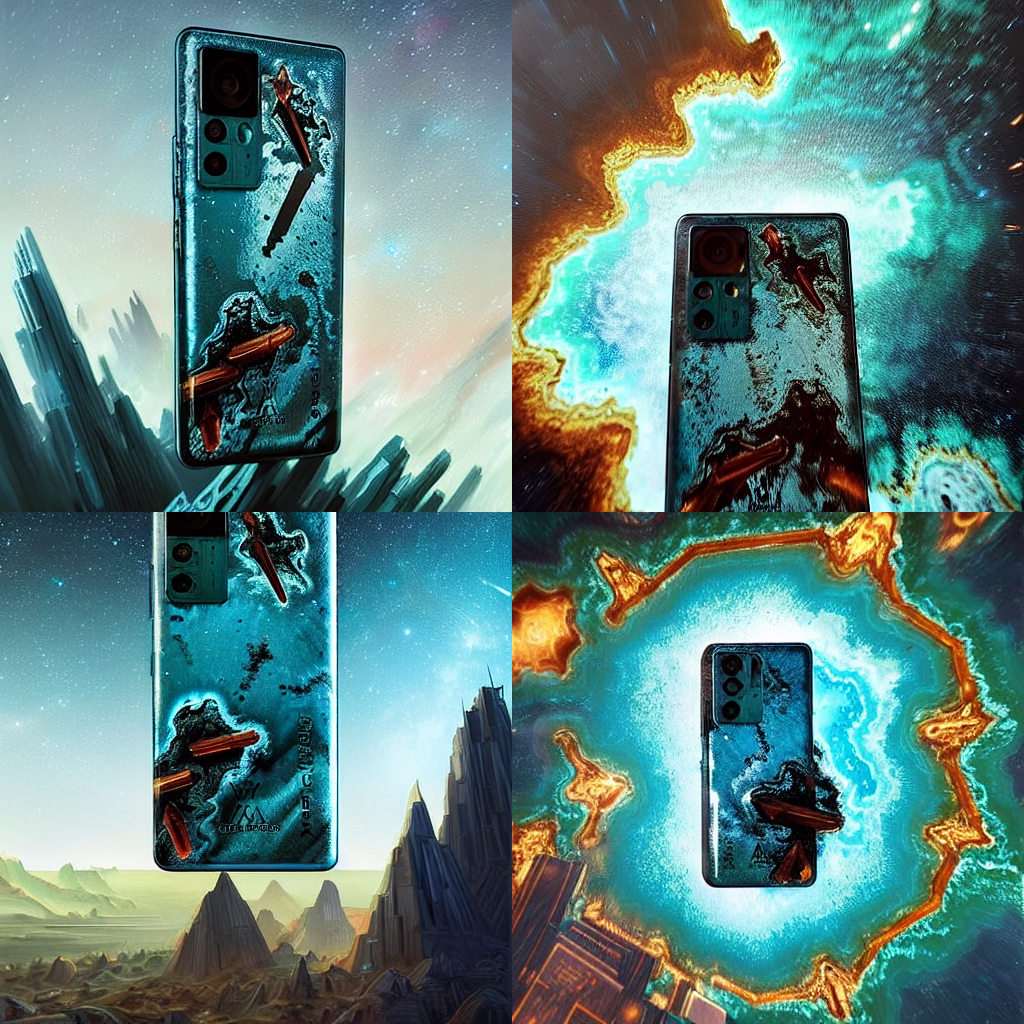
3D scene editing has entered the era of AI text interaction
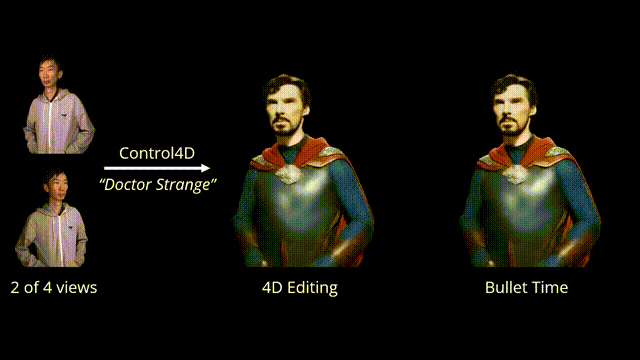
Works Series - Dimensional Recasting
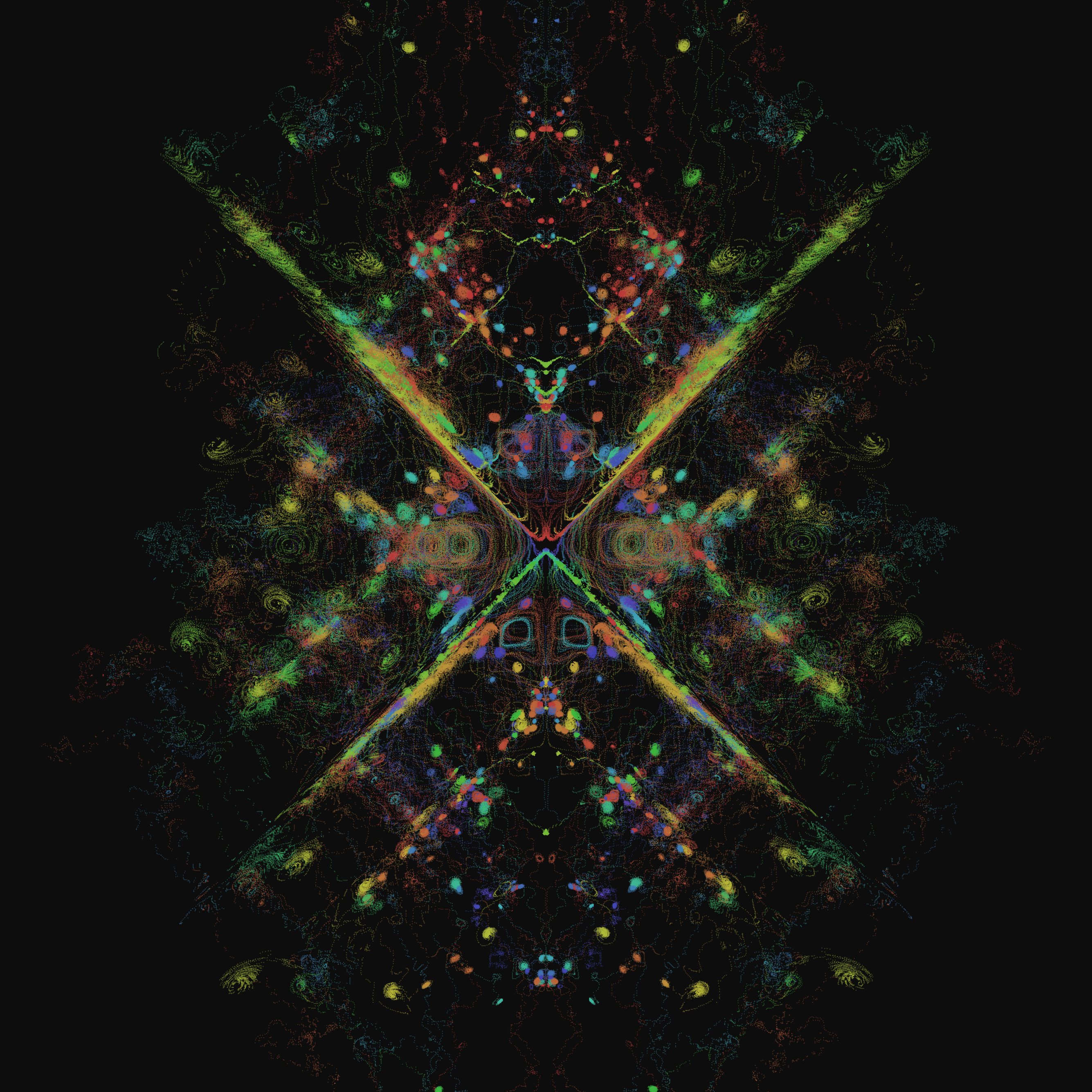
The Basic Principles of ChatGPT
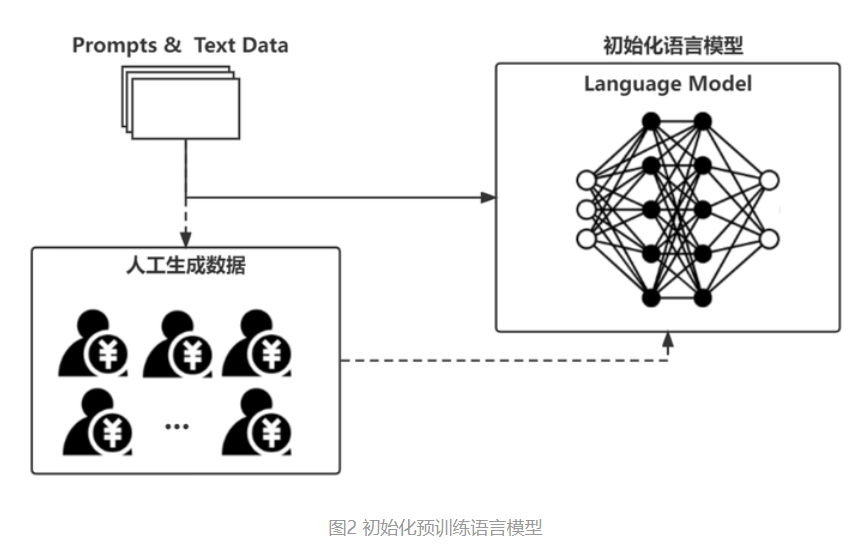
From Hand Modeling to Text Modeling: A Comprehensive Explanation of the Latest AI Algorithms for Generating 3D Models from Text
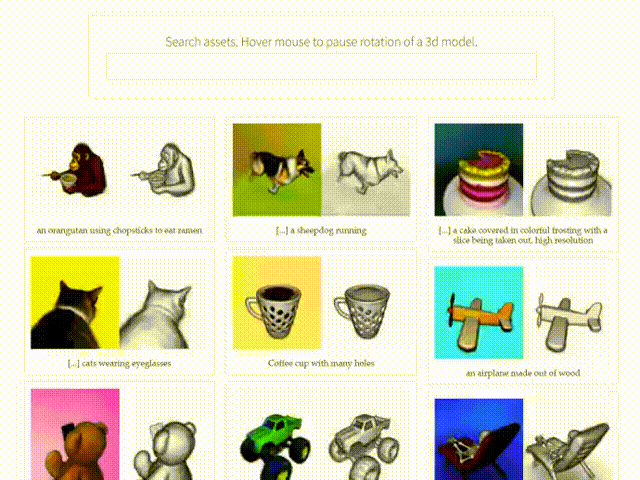